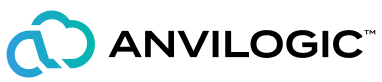
2025
State of
Detection Engineering
Perspectives from security practitioners and managers at various stages of their detection engineering journey.
Table of Content
Foreword
Mission Intel: Key findings
Methodology and participant demographics
1
Chapter 1: The rise of detection engineering
2
Chapter 2: Leadership support and key metrics
3
Chapter 3: Most valuable skills
4
Chapter 4: Implementing effective detections
5
Chapter 5: Black holes in data management
6
Chapter 6: The future of detection engineering
Actionable takeaways
Final thoughts
Book a Demo
Detection engineering has evolved from niche to necessity.
As someone who has built and scaled detection engineering teams over the years, I've witnessed this field evolve from a specialized skillset reserved for only the top 1% of security teams, to a mission-critical function that many organizations are now investing in. Throughout this journey, one truth has remained constant: organizations that invest in detection capabilities consistently demonstrate stronger security postures than those that don't. Yet the path to effective detection engineering remains challenging for many security teams.
Anvilogic’s inaugural 2025 State of Detection Engineering Report, in partnership with SANS Institute, highlights a fast-evolving field many organizations recognize as critical to modern cybersecurity. Based on responses from 264 security experts with detection engineering responsibilities across various regions, industries, and company sizes, the survey reveals how detection engineering (DE) has gained board-level attention yet faces resource, data, and skill challenges.
The data shows a pressing need for robust data management, specialized skills, and strategic leadership alignment to meet evolving threats head-on. While most organizations in our survey are investing in detection engineering, only about half have dedicated teams. Larger enterprises tend to allocate more staff, but many mid-sized and smaller companies still embed detection engineering responsibilities within other security roles.
There’s a paradox at play: leadership support is strong, with some CISOs even championing detection metrics at the board level. Yet despite this high-level buy-in, many teams still lack access to the right data feeds necessary for effective threat detection. Collecting, normalizing, and integrating security data remains one of the biggest operational challenges.
The shift to behavior-based detections, considered the most effective in the survey, also reveals skill gaps in threat modeling and data engineering. Many teams lack the expertise to embrace this proactive, analytics-driven approach fully. Confidence in detecting common threats like ransomware is high, but difficulty obtaining relevant logs and ongoing issues with tool misconfigurations and false positives suggest organizations remain vulnerable to advanced threats.
AI and automation are on the rise to help alleviate these challenges. Many already use AI in detection engineering, and most believe AI will significantly impact the field in the next few years. Automation is similarly ascendant, with most integrating automated workflows and more planning to do so.
What this all means is that detection engineering is no longer just an operational task—it’s becoming a defining pillar of security resilience. As threats grow more sophisticated, we can’t rely solely on vendor-provided detections and manual triage. Formalizing teams, closing data gaps, upskilling for the future, and carefully embracing automation and AI are key to moving from ad hoc efforts to a disciplined, programmatic approach.
With quantative survey data and authentic voices from our detection engineering community, we hope this report provides the insights and inspiration to help you refine your detection engineering strategy, elevate your team’s capabilities, and drive the field forward—together.
Anvilogic’s inaugural 2025 State of Detection Engineering Report, in partnership with SANS Institute, highlights a fast-evolving field many organizations recognize as critical to modern cybersecurity. Based on responses from 264 security experts with detection engineering responsibilities across various regions, industries, and company sizes, the survey reveals how detection engineering (DE) has gained board-level attention yet faces resource, data, and skill challenges.
The data shows a pressing need for robust data management, specialized skills, and strategic leadership alignment to meet evolving threats head-on. While most organizations in our survey are investing in detection engineering, only about half have dedicated teams. Larger enterprises tend to allocate more staff, but many mid-sized and smaller companies still embed detection engineering responsibilities within other security roles.
There’s a paradox at play: leadership support is strong, with some CISOs even championing detection metrics at the board level. Yet despite this high-level buy-in, many teams still lack access to the right data feeds necessary for effective threat detection. Collecting, normalizing, and integrating security data remains one of the biggest operational challenges.
The shift to behavior-based detections, considered the most effective in the survey, also reveals skill gaps in threat modeling and data engineering. Many teams lack the expertise to embrace this proactive, analytics-driven approach fully. Confidence in detecting common threats like ransomware is high, but difficulty obtaining relevant logs and ongoing issues with tool misconfigurations and false positives suggest organizations remain vulnerable to advanced threats.
AI and automation are on the rise to help alleviate these challenges. Many already use AI in detection engineering, and most believe AI will significantly impact the field in the next few years. Automation is similarly ascendant, with most integrating automated workflows and more planning to do so.
What this all means is that detection engineering is no longer just an operational task—it’s becoming a defining pillar of security resilience. As threats grow more sophisticated, we can’t rely solely on vendor-provided detections and manual triage. Formalizing teams, closing data gaps, upskilling for the future, and carefully embracing automation and AI are key to moving from ad hoc efforts to a disciplined, programmatic approach.
With quantative survey data and authentic voices from our detection engineering community, we hope this report provides the insights and inspiration to help you refine your detection engineering strategy, elevate your team’s capabilities, and drive the field forward—together.
Mission intel: Key findings
Detection engineers are some of the unsung heroes of cybersecurity — the builders, the problem-solvers, the ones turning chaos into clarity. They work tirelessly to craft, tune, and scale the detections, needed to keep organizations safe from a constantly evolving threat landscape.
At Anvilogic, we believe their work deserves more than just recognition — it deserves a spotlight. In partnership with the SANS Institute, we set out to gather intel straight from the people pushing the boundaries of detection engineering in our community. Here’s what we uncovered:
At Anvilogic, we believe their work deserves more than just recognition — it deserves a spotlight. In partnership with the SANS Institute, we set out to gather intel straight from the people pushing the boundaries of detection engineering in our community. Here’s what we uncovered:
80% of surveyed detection engineers said their organizations are putting real money behind DE
The majority of detection engineers reported that their organizations are actively funding detection engineering, with investment rising to 85% among large enterprises (5,000+ employees). The takeaway is clear: detection engineering isn’t just being adopted—it’s becoming a strategic priority.
From tactical to strategic: custom behavioral detections take the lead
Organizations are shifting from tactical alerting relying mostly on vendor-provided rules to strategic, custom-built detections. The top detection type preferred is behavior-based (67%), and custom-derived detections were the most common source (42%). Only 2% relied solely on vendor-provided detections. As detection engineering matures, threat modeling (53%) has emerged as a key skill for teams looking to level up.
Automation is thriving, AI is arriving
Participants overwhelmingly believe AI will play a major role in detection engineering (88% in the next three years), and today, 45% of organizations have already integrated AI into their detection workflows. Automation adoption shows stronger momentum, with 93% of organizations using or planning to implement automation in their workflows.
Leadership support is strong, but understanding still lags
Most detection engineers (67%) reported strong leadership buy-in, with some even saying it’s viewed as “the future” of security. For those without strong backing, the main reason is clear: detection engineering is still misunderstood in some organizations. The takeaway? Education and communicating the ROI to leaders will be key in closing the gap.
Data access and quality remain a key challenge
Detection engineering is only as strong as the data that fuels it. But for many teams, access and quality remain major obstacles. Our survey revealed a near-even split between those with adequate data access and those hitting roadblocks that limit their detection capabilities. Data engineering (52%) is now a top skill gap that detection teams are looking to close.
Methodology and participant demographics
To get an authentic voice of detection engineers, we went straight to the source, leveraging our partnership with the SANS Institute to tap 264 security professionals across diverse industries, regions, and organization sizes.
Before we dive in, it’s important to note that the findings in this report reflect insights from security experts actively involved in detection engineering. To qualify as a participant, respondents had to hold detection engineering responsibilities at their current organization. As a result, organizations without any detection engineering practices are not included in this data.
Before we dive in, it’s important to note that the findings in this report reflect insights from security experts actively involved in detection engineering. To qualify as a participant, respondents had to hold detection engineering responsibilities at their current organization. As a result, organizations without any detection engineering practices are not included in this data.
What is your primary role in the organization, whether as an employee or consultant?
Practitioner
Executive
Management
Other
What is your company's primary industry?
22%
Cybersecurity
17%
Technology
14%
Banking and finance
8%
Healthcare
8%
Other
6%
Government
5%
Education
5%
Manufacturing
4%
Telecommunications/ISP
3%
Retail
In what country or region is your primary corporate headquarters?
United States
Europe
Canada
Asia
Other
How large is your organization’s workforce, including both employee and contractor staff?
Under 5k Employees
Over 5k Employees
Chapter 1
The rise of detection engineering
Just a decade ago, detection engineering was a relatively unknown role in cybersecurity. Now, it’s emerging as one of the most critical roles in security operations, with most organizations in the survey having dedicated detection engineering teams.
Defining detection engineering
Chances are you picked up this report because you already have an idea, but what’s the true definition of detection engineering? SANS Institute and Anvilogic agreed on the definition below.
“Detection engineering is the systematic and iterative process of developing, implementing, testing, deploying, and maintaining high-fidelity threat detection mechanisms. It is a specialized field that combines elements of software engineering, data analysis, security operations, and threat intelligence to build and maintain a robust detection capability. Unlike traditional security approaches that often rely on pre-configured, vendor-provided detection rules, detection engineering emphasizes the development of custom, context-aware detections tailored to the organization's specific environment, assets, and threat landscape.”
What are the primary activities related to detection engineering in your organization?
86%
Developing detection rules
Most detection engineers focus on developing new detection rules, highlighting that organizations place an importance on building detection content customized to their unique environment.
82%
Tuning existing detections
This high percentage suggests that many teams are caught in a cycle of manually adjusting and fixing detection rules that may be broken or ineffective, highlighting a potential need for more efficient, automated approaches to detection management.
61%
Automation and orchestration
58%
Incident response
58%
Threat hunting
55%
Threat intelligence integration
51%
Metrics and reporting
50%
Security tool administration
49%
Training and knowledge sharing
39%
Data engineering for security
3%
Other
“Any job in cybersecurity involves some level of complexity hence the use of analogies. I believe Detection Engineering and Security Analytics practices resemble culinary arts. The logs are the ingredients; the menu, the detections and analytics; and the main guest is Security Operations. The Detection Engineer acts like a cook. The craft of turning raw ingredients into something more digestible and refined can be very well rewarded, especially in times of data abundance and alert fatigue. That said, if you don't taste your own food, you risk serving poor dishes. If you have already worked as a Security Analyst in a SOC before, it makes it easier for you to thrive as a Detection Engineer.
Today it's not only about knowing OS internals or Cloud technology, it involves data fluency and understanding the attacker mindset (offensive security). However, if I am recruiting today, I look for query language fluency, whether it's SPL, KQL or even SQL. That's the "Mise en place" you must know in order to manipulate data and work in my kitchen.
And here's where Data and Detection Engineering slightly overlap. Log data need to be collected, sorted and understood before being consumed in the detection pipeline. In the end, a successful Detection Engineer not only needs to know how to prepare and treat data, but also needs to know how to make sense of it from a business and security perspective.”
Today it's not only about knowing OS internals or Cloud technology, it involves data fluency and understanding the attacker mindset (offensive security). However, if I am recruiting today, I look for query language fluency, whether it's SPL, KQL or even SQL. That's the "Mise en place" you must know in order to manipulate data and work in my kitchen.
And here's where Data and Detection Engineering slightly overlap. Log data need to be collected, sorted and understood before being consumed in the detection pipeline. In the end, a successful Detection Engineer not only needs to know how to prepare and treat data, but also needs to know how to make sense of it from a business and security perspective.”
Job Titles: A diverse and evolving field
As detection engineering has skyrocketed in importance, so has the variety of job titles associated with it. Many security practitioners juggle detection engineering alongside other responsibilities—such as threat hunting and incident response—so it’s no surprise that titles vary widely. Some of the job titles we came across while doing this survey are reflected in the chart below.
Individual Contributor Job Titles
- Detection Engineer (most common)
- Threat Hunter
- Security Engineer
- Security Researcher
- Cloud Security Engineer
- Security Architect
- CSIRT Team Lead
- Incident Response Engineer
- Threat Intelligence Analyst
- Cloud Security Researcher
- Intrusion Analyst
- SIEM Specialist
- Senior Security Analyst
Leadership Job Titles
- CISO
- VP Data Science
- VP of Security Services
- VP of Cyber Operations
- Director of Security Operations
- Director, Threat Detection
- Director of Detection Engineering
- Director of Information Security
- SOC Manager
- Detection Engineering Manager
- Security Engineering Manager
- Cyber Defense Analytics Manager
- Cyber Incident Response Manager
An advanced field still developing senior talent
Detection engineering has come a long way, but the talent pool is still evolving. While the field itself has matured beyond its early days, most detection engineers fall into the mid-career range, with fewer senior-level professionals. As demand for expertise rises, investing in mentorship, training, and career development will be essential to closing the skills gap and growing the next generation of senior talent.
On average, how many years of experience do
your workforce members have in detection engineering?
your workforce members have in detection engineering?
1-2
3-5
6-10
11+
Unknown/Unsure
None
“Experience is particularly important in detection engineering because it can take years to complete the feedback loop of designing, maintaining, and testing a detection's performance against adversary activity (emulated or real). Poorly designed or implemented detections are easy to produce but do little to increase the probability of detecting an adversary. Detection modeling and purple team testing are critical efforts that shorten the feedback loop and build experience in what is still a relatively young discipline.”
60% of detection engineers work on a dedicated team
Many organizations are prioritizing detection engineering as its own function, with 60% maintaining dedicated teams across all company sizes. This commitment is more pronounced in enterprises with over 5,000 employees, where 70% have established dedicated detection engineering teams. In contrast, small and medium-sized organizations with fewer than 5,000 employees report that only 49% have dedicated engineering teams.
Do you have a dedicated team for detection engineering?
Yes
No
Unsure
SMB Organizations
Up to 5,000 Employees
Up to 5,000 Employees
Enterprise Organizations
More Than 5,000
More Than 5,000
Enterprises are investing heavily in detection engineering talent
As anticipated, larger organizations are investing more heavily in detection engineering talent. 39% of enterprises (5,000+ employees) have six or more dedicated FTEs focused on detection engineering, compared to 27% in small and mid-sized organizations.
Indicate in terms of FTEs how many of your workforce
are doing detection engineering-type work?
are doing detection engineering-type work?
SMB Organizations
Up to 5,000 Employees
Up to 5,000 Employees
Enterprise Organizations
More Than 5,000
More Than 5,000
1-2
3-5
6-10
11+
None
Most put detection engineering as a dedicated team within security operations
When it comes to organizational structure, most organizations position their detection engineering function as a dedicated team within security operations, with over half (54%) taking this approach. A significant portion (27%) integrate their detection engineering team directly with their SOC teams, while only 10% distribute the role across multiple security operation teams.
Where does this team sit within your organization?
Separate team within security operations
Part of the SOC team
Distributed across multiple teams within security operations
Other
Part of IT operations
Teams detection engineers collaborate with the most
Detection engineers work most closely with incident response teams, with 58% reporting full integration and collaboration. Threat hunting teams follow closely behind at 48%. However, collaboration drops off when it comes to data engineering, infrastructure, and application teams—highlighting potential silos that could impact detection engineering program effectiveness.
How well integrated are your detection engineering efforts
with the following operational areas (e.g., teams)?
with the following operational areas (e.g., teams)?
Fully integrated and collaborative.
Partially integrated, with some collaboration.
Minimal integration, mostly independent.
Not integrated at all.
Chapter 2
Leadership support and key metrics
Detection engineers from our survey report that their organization is significantly investing in the function, driven by strong executive support. Security teams measure the effectiveness of their detection efforts using key metrics such as accuracy, threat coverage, and detection speed, which many report out on at the board-level.
80% reported their organization is actively investing in detection engineering
Detection engineering is an investment priority for organizations who participated in our survey with 80% reporting they are actively investing in building this capability—regardless of company size. Enterprises (5,000+ employees) are even more committed, with 85% funding detection engineering initiatives.
Is your organization currently investing in detection engineering?
SMB Organizations
Under 5K Employees
Under 5K Employees
Yes, we are currently investing in detection engineering.
No, we are not investing nor are we planning to.
Unknown/Unsure.
Enterprise Organizations
Over 5K Employees
Over 5K Employees
All Data
“Detection engineering has evolved from a niche function to a critical security priority, with a lot of organizations investing in it. The shift from signature-based to behavior-based detection highlights a need for better data access and specialized skills. Challenges remain in collecting and integrating data effectively. Over the next three years, AI and automation will drive detection capabilities, while formalized teams and improved data management will enhance efficiency. Organizations must align detection strategies with business goals to stay ahead of evolving threats.”
"Detection Engineering is at the forefront of proactive cybersecurity, influencing organizations’ security postures. Organizations have recognized the strategic advantage of detection engineering as a security function, with nearly 80% of survey respondents indicating that their organizations are actively investing in this critical security function. The skills and experience of detection engineers are instrumental in shaping a company’s security organization."
"We need to realize that a busy SOC is not always an efficient one, especially not when it is drowning in ever more dubious alerts, incapable of measuring its actual detection coverage and let alone extend it. Automating IR is valued, but doesn’t fix the core problem: we need better detections.
Instigating detection engineering programs enable teams to finally target repeatable intelligence-to-detections workstreams, optimize the entire alert lifecycle, and ultimately transform SOCs from costly alert processors to highly informed threat detection organizations. Detection engineering isn’t just a nice-to-have, it’s a need to manage a performance SOC."
Instigating detection engineering programs enable teams to finally target repeatable intelligence-to-detections workstreams, optimize the entire alert lifecycle, and ultimately transform SOCs from costly alert processors to highly informed threat detection organizations. Detection engineering isn’t just a nice-to-have, it’s a need to manage a performance SOC."
67% feel there is strong leadership support for detection engineers
We asked survey respondents whether their leadership supports detection engineering initiatives—and the responses were both encouraging and revealing. Two-thirds (67%) report strong executive buy-in, with some CISOs championing detection efforts at the board level.
However, not all teams feel the same level of support. Some leaders fail to fully understand the complexity and strategic value of detection engineering, leading to misalignment, resource challenges, or a lack of dedicated roles. In some cases, detection teams were left out of key technology decisions or struggled to gain cross-functional collaboration for data access and integration.
However, not all teams feel the same level of support. Some leaders fail to fully understand the complexity and strategic value of detection engineering, leading to misalignment, resource challenges, or a lack of dedicated roles. In some cases, detection teams were left out of key technology decisions or struggled to gain cross-functional collaboration for data access and integration.
Is there strong leadership support for
detection engineers at your organization?
detection engineers at your organization?
Yes
No
Unknown/Unsure
“The most valuable support executives can provide for a detection engineering program is investing in the right tools and ensuring full visibility into company data—especially through strong audit logging. Clear, high-quality log data is essential because it allows detection engineers to spot attack patterns, build effective detections, and fine-tune them over time. Without reliable telemetry, the team's ability to detect and respond to threats is severely limited.
Detection engineering teams can demonstrate their value within the security department by sharing their core security expertise and custom tooling, extending their impact beyond detection rule writing. By improving visibility into company telemetry, they directly enhance incident response teams’ ability to detect and respond to threats. Additionally, their deep understanding of attack patterns allows them to support cloud security, infrastructure, and other teams in threat modeling and strengthening system defenses.”
Detection engineering teams can demonstrate their value within the security department by sharing their core security expertise and custom tooling, extending their impact beyond detection rule writing. By improving visibility into company telemetry, they directly enhance incident response teams’ ability to detect and respond to threats. Additionally, their deep understanding of attack patterns allows them to support cloud security, infrastructure, and other teams in threat modeling and strengthening system defenses.”
We also invited security professionals to share their experiences anonymously, offering an authentic look at how leadership support (or the lack of it) impacts them. Here’s what they had to say—in their own words.
Most common metrics for measuring detection engineering success
What metrics do you use to measure
the effectiveness of your detection engineering efforts?
the effectiveness of your detection engineering efforts?
70%
False positive rate
Not only does a high false positive alert distract teams from missing a real threat, but it also causes a mountain of work and additional costs downstream for SOC analysts who have to triage and investigate alerts.
60%
Improved threat coverage
Expanding detection coverage for the threat priorities unique to your environment demonstrates the detection engineering team is reducing blind spots and minimizing risk. Some survey respondents indicate this metric often reaches board-level visibility, with their CISOs regularly reporting on it.
59%
Coverage of attack techniques
Mapping detections to frameworks like MITRE ATT&CK ensures visibility across the attack lifecycle and increases the likelihood of catching adversaries.
54%
Detection speed
Fast deployment of new detection rules in response to emerging threats or red team findings reduces risk by quickly closing detection gaps. In a recent report from Anvilogic and ESG, 86% of security professionals said it takes a week or more (i.e., identifying the need, creating the detection, testing, and deploying the detection).
32%
Reduction in incident response time
32%
Incident response time
31%
Reduction in successful attacks
23%
Size of detection engineering backlog (specify number of rules to build)
4%
Other
“Achieving long term support for DE requires expanding our value proposition. DE should not mean content only. These programs are best utilized as a force multiplier engine in fusion center operating models. Use evaluations based on impact instead of MITRE ATT&CK coverage. Measure the program by how fast, accurate, and complete SecOps teams have a timeline of threat events, and actor enrichment before triage. You can increase the program's CMMI by investing in exploit research, automation, and data science activities. Operate with a culture of experimentation in mind, and include AI agents in your strategy.”
*Disclaimer: The views and opinions expressed by Dennis Chow here are solely his own and do not reflect his employer’s official policy or position.
*Disclaimer: The views and opinions expressed by Dennis Chow here are solely his own and do not reflect his employer’s official policy or position.
Chapter 3
Most valuable skills
Overall, detection engineering teams are feeling good about their understanding of attack frameworks and their triaging and incident response, but they also recognize some areas where additional skills are sorely needed. Threat modeling and data engineering top the list of critical skills that still need development in detection engineering.
The top most-enjoyed activity identified among detection engineers was developing detection rules, so it looks like they’re in the right job. But when it comes to metrics and reporting? Let’s just say it’s not a fan favorite. Detection engineers overwhelmingly dislike it—and honestly, we’re not surprised.
The top most-enjoyed activity identified among detection engineers was developing detection rules, so it looks like they’re in the right job. But when it comes to metrics and reporting? Let’s just say it’s not a fan favorite. Detection engineers overwhelmingly dislike it—and honestly, we’re not surprised.
Essential Detection engineering skills:
What’s locked in & what needs work
What skills are most valuable for your detection engineering workforce?
Indicate those you currently have, those that need development, and those
that are not applicable.
Indicate those you currently have, those that need development, and those
that are not applicable.
Current
76%
Understanding/ mapping
attack frameworks
attack frameworks
This reflects the organizations’ strong adoption of frameworks like MITRE ATT&CK for threat detection and security program development.
74%
Triage & incident response
As the second most valuable skill set, it demonstrates that detection engineering teams maintain strong operational security skills essential for effective threat response.
67%
Processing/ querying languages (e.g. SPL, SQL, KQL)
61%
Regular expressions
60%
Threat intelligence /
research analysis
research analysis
54%
Documentation
48%
Scripting/ programming languages (e.g. Python, JavaScript)
48%
Alert enrichment (before or after an alert is generated)
46%
Reporting/ visualizations
43%
Log pipeline monitoring and health
38%
Threat Modeling
36%
Detection-as-code, CI/CD
35%
Data engineering
23%
Software engineering
2%
Other
Need Development
53%
Threat modeling
Teams are recognizing the importance of proactive security architecture and attack path mapping.
52%
Data engineering
This highlights the growing need for security professionals who can effectively manage and analyze large security datasets.
47%
Reporting/ visualization
This indicates that teams struggle to communicate their findings and metrics to key
stakeholders effectively.
stakeholders effectively.
47%
Software engineering
46%
Detection-as-code, CI/CD
45%
Log pipeline monitoring and health
45%
Alert enrichment (before or after an alert is generated)
44%
Scripting/ programming languages
(e.g. Python, JavaScript)
(e.g. Python, JavaScript)
42%
Documentation
36%
Threat intelligence /
research analysis
research analysis
32%
Regular expressions
27%
Processing/Querying Languages (e.g. SPL, SQL, KQL)
21%
Understanding/ mapping attack frameworks (e.g. MITRE ATT&CK)
19%
Triage & incident response
3%
Other
“Threat modeling identifies potential security risks and designs mitigations early in the development cycle, providing a proactive approach to managing potential threats. For detection engineers, frameworks such as MITRE ATT&CK and STRIDE provide structured guidance for creating and refining detection rules.
However, this critical skill is often neglected due to limited training opportunities and reliance on reactive measures. Challenges include understanding complex systems, aligning security with business goals, and seamlessly integrating modeling into workflows, as there is no one-size-fits-all approach to threat modeling. By using tools based on these frameworks and conducting regular reviews, organizations can effectively address these issues and enhance their security posture.”
However, this critical skill is often neglected due to limited training opportunities and reliance on reactive measures. Challenges include understanding complex systems, aligning security with business goals, and seamlessly integrating modeling into workflows, as there is no one-size-fits-all approach to threat modeling. By using tools based on these frameworks and conducting regular reviews, organizations can effectively address these issues and enhance their security posture.”
“It’s not surprising that so many respondents reported that data engineering is a missing skill set – “data engineering” is an emerging subdomain within security operations and most existing solutions were built to solve observability use cases, not security use cases. Security data engineering solutions need to solve the trifecta of data routing, data normalization, and data enrichment across dozens of unique and disconnected systems, and observability tools – which are primarily built to route and summarize data for applications – are not the best solutions to these problems.”
Detection-as-Code emerges to scale detections without the headaches
Detection engineers didn’t sign up to babysit security tools. They thrive on mapping threats, building smarter detections and staying ahead of attackers—not getting stuck in an endless loop of version iterations, rule maintenance, and configuration headaches. Managing thousands of detections at scale is a beast without applying software engineering principles to the detection management lifecycle, especially when each change introduces additional layers of tuning complexities.
Enter Detection-as-Code (DaC) with 46% of detection engineers identifying it as a skill they need to develop, closely mirroring the 47% who see software engineering skills as an area for growth. We’ve seen growing adoption of DaC in various forms, but at its core, it’s a remedy for detection maintenance fatigue and a way to scale custom detection development. It frees engineers from the mind-numbing grind of upkeep, and lets them focus on what actually excites them: solving complex security puzzles and translating threat behaviors into actionable detections. Because let’s face it—nobody gets into security to babysit rules all day.
Enter Detection-as-Code (DaC) with 46% of detection engineers identifying it as a skill they need to develop, closely mirroring the 47% who see software engineering skills as an area for growth. We’ve seen growing adoption of DaC in various forms, but at its core, it’s a remedy for detection maintenance fatigue and a way to scale custom detection development. It frees engineers from the mind-numbing grind of upkeep, and lets them focus on what actually excites them: solving complex security puzzles and translating threat behaviors into actionable detections. Because let’s face it—nobody gets into security to babysit rules all day.
“The shift to Detection-as-Code (DaC) highlights a growing need for detection engineers to merge security expertise with software engineering skills. While current skills focus on analysis and threat detection, future demands include version control, automated testing, and CI/CD proficiency. Bridging these gaps requires upskilling in tools and scripting languages, as well as fostering collaboration between security and engineering teams.
By adopting software development practices, organizations can build scalable, consistent, and efficient detection programs, empowering teams to collaborate more effectively, pass knowledge more efficiently, and maintain greater confidence in their detection capabilities.”
By adopting software development practices, organizations can build scalable, consistent, and efficient detection programs, empowering teams to collaborate more effectively, pass knowledge more efficiently, and maintain greater confidence in their detection capabilities.”
“I'm delighted to see respondents recognize the importance of software engineering and detection-as-code as a way to scale their program.
SRE/DevOps/Software Engineers have built juggernaut products that seem like it took an army
of engineers to build, but due to their focus on scaling with software, have kept headcount at a growth rate that is 10x less than the software they have built.
Writing detections and finding badness should always be the goal, but we should continue learning tools and technologies built by software engineers and DevOps to deal with finding threat actors inside the massive amounts of telemetry we deal with everyday.”
SRE/DevOps/Software Engineers have built juggernaut products that seem like it took an army
of engineers to build, but due to their focus on scaling with software, have kept headcount at a growth rate that is 10x less than the software they have built.
Writing detections and finding badness should always be the goal, but we should continue learning tools and technologies built by software engineers and DevOps to deal with finding threat actors inside the massive amounts of telemetry we deal with everyday.”
“Detection Engineering is evolving from deploying detections in vendor-specific consoles using proprietary languages to adopting more scalable methodologies, such as Detection-as-Code (DaC). This mature approach incorporates Software Development Life Cycle (SDLC) and Continuous Integration/Continuous Deployment (CI/CD) practices, which are areas of expertise for many software engineers.
As large language models (LLMs) and AI agents gain traction in security operations, the demand for data engineering and software engineering skills will continue to grow, further expanding the skill set required for detection engineers.”
As large language models (LLMs) and AI agents gain traction in security operations, the demand for data engineering and software engineering skills will continue to grow, further expanding the skill set required for detection engineers.”
Top activities detection engineers enjoyed the most and least
Which detection activities do you, as a professional, enjoy the most? The least?
Most
33%
Developing detection rules
Most detection engineers are fulfilled by creative problem-solving and the technical challenge of translating threat behaviors into actionable detections.
19%
Threat hunting
Detection engineers often call this the “fun” work which involves the investigative aspects of discovering new threats and attack patterns.
11%
Incident response
Considering detection engineers reported the highest collaboration with the incident response team, it's no surprise that they enjoy this aspect of the job.
9%
Automation and orchestration
4%
Data engineering for security
4%
Data engineering for security
3%
Security tool administration
3%
Training and knowledge sharing
3%
Tuning existing detections
1%
Metrics and reporting
1%
Other
Least
41%
Metrics and reporting
This likely stems from the political alignment needed to agree on metrics and the tedious nature of data collection, dashboard creation, and regular status reporting, which takes time away from more technical and creative detection work.
10%
Security tool administration
Detection engineers prefer to focus on building detections and threat hunting rather than dealing with security tools that require a ton of maintenance, configurations, and platform management.
9%
Tuning existing detections
While this occupies most of the detection engineers’ time and is generally accepted as a valuable part of their role, it is still time-consuming, manual, and tedious.
No wonder it’s a least favorite!
No wonder it’s a least favorite!
9%
Data engineering for security
While low on the favorite list of activities, this represents a critical skills gap that detection engineering teams need to address.
9%
Incident response
4%
Developing detection rules
4%
Automation and orchestration
3%
Threat hunting
3%
Threat intelligence integration
1%
Other
“To manage competing priorities of creative and operational tasks, we break them into chunks. One thing my organization tries to do is hold "fun Fridays" where we all get together and pause our operational tasks to focus on something we consider fun, such as testing a detection idea, deploying a new detection, or researching a potential attack path. This improves morale, allows us to stay up to date with the industry, breaks apart more monotonous operational tasks, and exercises a different part of our brains while allowing us to come back to the operational tasks with a fresh outlook.”
“Operational tasks, though less exciting, still hold significant value and can present opportunities to learn or enhance skills. For instance, automating reporting through Detection-as-Code or leveraging data analysis libraries can streamline the report generation process, making it nearly effortless.
As for tuning, when possible, I recommend empowering analysts to address tuning requests using novel problem-solving skills. Analysts often possess valuable context about noisy detections and can make significant contributions to enhancing analytic capabilities. Reframing operational tasks as opportunities to improve workflows, acquire new skills, and foster collaboration makes these tasks less tedious and far more impactful!”
As for tuning, when possible, I recommend empowering analysts to address tuning requests using novel problem-solving skills. Analysts often possess valuable context about noisy detections and can make significant contributions to enhancing analytic capabilities. Reframing operational tasks as opportunities to improve workflows, acquire new skills, and foster collaboration makes these tasks less tedious and far more impactful!”
Most commonly used tools and technologies
Which tools/technologies do you regularly use for detection engineering?
Open Source
58%
Custom scripts/ tools
30%
Threat intelligence platforms
30%
Jupyter notebooks
27%
Version control systems
20%
CI/CD pipelines
15%
SIEM platforms
12%
Data lakes
12%
Kubernetes security tools
10%
Cloud security tools
9%
SOAR tools
8%
MDR solution
8%
EDR/XDR solutions
2%
Other
Product Based
89%
EDR/XDR solutions
81%
SIEM platforms
70%
Cloud security tools
69%
SOAR tools
64%
Threat intelligence platforms
61%
Data lakes
60%
MDR solution
47%
Version control systems
42%
CI/CD pipelines
41%
Kubernetes security tools
24%
Custom scripts/tools
14%
Jupyter notebooks
2%
Other
Chapter 4
Implementing effective detections
A strong preference for behavior-based detection methods over traditional approaches in our survey responses indicates a shift towards more sophisticated and adaptable detection strategies. Custom-derived detections are the most commonly reported source of detections (this is a report about detection engineers, afterall), but organizations also use vendor-provided and open-source detections.|
Behavioral-based is the top-ranked type of detection found to be most effective
What are the top three detection types you find most effective?
67%
Behavior-based
It’s clear that analyzing patterns and deviations in system behavior is emerged as the preferred detection type, providing superior detection of novel threats and attack techniques that evade traditional approaches.
43%
Correlation-based
Teams correlate multiple data points and alerts to detect more complex attack patterns, providing a more comprehensive view of potential threats.
43%
Threat inteligence-driven
Many organizations use real-time threat feeds and indicators to strengthen detection capabilities, but cost, integration complexity, and rapid obsolescence can limit adoption and strain resources.
41%
Signature-based
34%
Anomaly-based
20%
User and entity behavior analytics (UEBA)
19%
Network traffic analysis
14%
Machine learning-powered
10%
Cloud-specific
8%
Asset-centric
2%
Other
“There's a clear growth element away from atomic detections into advanced detection types. Although signature and atomic detections can be precise with a low-false positive rate, they almost always are brittle and have poor recall. That is, creating a resilient detection, which is one that can account for changes in TTPs by threat actors, improves recall and can stand the test of time against an ever-evolving threat landscape. Layering detections and focusing on behaviors of threat actors can impose the most pain on them, forcing actors to change altogether to circumvent detections.”
“Behavior based detections resonate strongly with the surveyed community due to their adaptability and precision in real world scenarios. This preference aligns with a growing demand for detections that can interpret nuanced, environment specific activities. In practice, custom behavior-based detections have enabled faster identification of credential misuse or anomalous access patterns, particularly in Identity Access Management (IAM).
However, the challenges in implementing machine learning (ML) solutions often stem from their reliance on extensive historical data and potential for false positives. Teams should prioritize integrating hybrid approaches, leveraging behavior based models while iteratively refining ML for scalability and accuracy.”
However, the challenges in implementing machine learning (ML) solutions often stem from their reliance on extensive historical data and potential for false positives. Teams should prioritize integrating hybrid approaches, leveraging behavior based models while iteratively refining ML for scalability and accuracy.”
“Threats are always evolving, and security tools detect only as fast as they scan. Defenders must practice defense in depth and focus on behaviors of emerging threats. Blocking IOCs won’t suffice as they’re easily changed. Understanding the Pyramid of Pain explains why detection engineering is crucial. It highlights the importance of detecting threat behaviors, which are outlined in the MITRE framework. This framework helps beginners learn the attack chain and build effective detection queries.”
Most organizations update their detection rules and processes weekly or daily
Over half of detection engineers (56%) in our survey update their detection rules and processes daily or weekly, ensuring rules are tuned and relevant. However, 30% of organizations update rules only when needed, quarterly, or even annually.
How often do you update your detection rules and processes?
Daily
Weekly
Monthly
Only when needed
Quarterly
Unknown/Unsure
Annually
Custom detections lead the way
We asked survey participants to break down what sources they use on average for detection rules. Most detections (42%) were custom-built to fit their organization’s unique environments. Vendor-provided detections come in second at 37%, but few rely on them exclusively. Only 2% use vendor detections alone and 15% don’t use vendor-provided detections at all. 6% of participants don’t use any custom-derived detections and 32% don’t use any open-source detections.This highlights a clear preference for tailored, organization-specific detections.
What percentage of your detections are from the following sources?
Custom derived
Vendor provided
Open Source
Unknown
“Detections provided by security vendors are valuable but must cater to a broad customer base. CISOs and SOC managers are recognizing the need to develop targeted detections aligned with their specific threat models, fine-tuned to their unique environments, using their visibility, and focused on their most significant threats. This has led many organizations to create thousands of detections that go beyond atomic indicators and basic signatures, requiring dedicated analysts to manage their creation, maintenance, organization, and testing.”
Top benefits of building custom detections
What are the main benefits you see in creating your own detections?
81%
Greater accuracy tailored to our environment
72%
More control over detection logic
67%
Ability to innovate and test new ideas
39%
Faster response to new threats
2%
Other
“The importance of building your own custom detections in lieu of relying solely on vendor detections is directly proportional for the business's reliance on the comprehension of their own environment. Vendors and their technology stack are great tools for businesses to use to understand their industry sector and neighboring sectors but no vendor driven or installed solution will ever be able to adequately analyze and explain your environment or it's practices effectively.
A common scenario in the modern threat landscape is abuse of the living off the land techniques specifically to avoid vendor driven detections, but an in-house team of detection engineers is able to understand the environment that they are in to create profiles and baselines of activity within the environment to better detect anomalies and malicious behavior(s) in otherwise innocuous applications.”
A common scenario in the modern threat landscape is abuse of the living off the land techniques specifically to avoid vendor driven detections, but an in-house team of detection engineers is able to understand the environment that they are in to create profiles and baselines of activity within the environment to better detect anomalies and malicious behavior(s) in otherwise innocuous applications.”
Top challenges of using vendor-provided detections
What challenges do you face when using vendor-provided detections?
64%
High false positive rate
61%
Issues with accuracy in our environment
54%
Lack of flexibility in customization
43%
Difficulty in understanding the logic behind detections
34%
Delays in updates or improvements
3%
Other
Top challenges of building and maintaining custom detections
What challenges do you encounter when building and maintaining custom detections?
71%
Resource and time constraints
53%
Complexity of threat landscape
49%
Difficulty in validating effectiveness
41%
Lack of skilled personnel
4%
Other
The top six areas detection engineering programs need the most improvement
We asked survey participants which areas of their detection engineering programs needed the most improvement and these six rose to the top of the list.
45%
listed ‘reducing false positives’ as an area needing improvement
Behavior-based detections are a go-to for detection engineers to tackle this problem, but many organizations struggle to fine-tune vendor-provided and custom detections as shifting payloads and missing properties make it harder to separate true signals from the noise.
43%
want to improve the ‘turnaround time for the entire process of developing and deploying a detection’
Teams still struggle to quickly turn detection concepts into deployed defenses, as manual, cumbersome processes create bottlenecks that slow progress. Meanwhile, the threat landscape evolves at an unforgiving pace. This growing gap between threat velocity and detection agility underscores the need for more streamlined, automated approaches.
39%
identified ‘automation of detection tasks’ as a critical need
Data shows that manual tasks consume hours each day—time working against detection engineers who are responsible for managing the detection lifecycle across numerous detections. Forward-thinking teams are exploring ways to automate repetitive tasks across maintenance, tuning, and implementation, allowing them to focus on higher-value work and apply their expertise where it matters most.
38%
of teams want to prioritize improving the ‘accuracy of detection rules’
Teams are stuck in a tightrope act—crafting rules precise enough to catch real threats while staying flexible enough to detect a wide range of techniques. This balancing act is driving them to adopt more sophisticated correlation-based detection strategies.
36%
emphasized ‘streamlining workflows’ as a key area for improvement
Teams are getting tangled in manual processes across multiple tools and UIs, with cumbersome maintenance slowing down detection deployment and updates. The need for smooth, intuitive workflows has never been more critical to maintaining & even boosting operational rhythm.
34%
pointed to improving ‘collaboration between teams’
Security doesn’t exist in a vacuum, but many detection engineering teams operate like they do—creating blind spots between security, IT, and business units. This disconnect limits access to critical data and stifles cross-functional breakthroughs. Breaking down these silos is more crucial than ever for a truly interconnected defense.
Chapter 5
Black holes in data management
Data access and quality emerge as significant hurdles
for many detection engineering teams.
This data bottleneck can severely limit the effectiveness of even the most sophisticated detection strategies, highlighting the need for improved data management practices across the industry.
for many detection engineering teams.
This data bottleneck can severely limit the effectiveness of even the most sophisticated detection strategies, highlighting the need for improved data management practices across the industry.
Nearly half reported inadequate access to the data needed for threat detection objectives
Only 45% reported having adequate data access for their threat detection objectives, underscoring a critical challenge. This presents an even greater issue for enterprise organizations, where 58% lack access or aren’t sure if they have the right logging in place to achieve their detection goals.
Do you feel you have adequate access to all of the data feeds/logging required
to meet your threat detection objectives?
to meet your threat detection objectives?
SMB Organizations
Up to 5,000 Employees
Up to 5,000 Employees
Yes
No
Unknown/Unsure.
Enterprise Organizations
More Than 5,000
More Than 5,000
All Data
“Detection engineering, especially with engineers that understand data and can build tooling and pipelines, is critical to finding and detecting attacks on various systems, including those considered “crown jewels” within the company.
One major challenge for detection engineers is securing the budget to store and retain the log data needed for effective detections and recursive threat hunting. Log storage costs can be significant, forcing organizations to make trade-offs between retention periods and collecting critical data sources.”
One major challenge for detection engineers is securing the budget to store and retain the log data needed for effective detections and recursive threat hunting. Log storage costs can be significant, forcing organizations to make trade-offs between retention periods and collecting critical data sources.”
“The survey shows that nearly half of organizations can't even get to their data, let alone use it for detection! So before we all go crazy with AI-powered threat detection, remember the golden rule: 'garbage in, garbage out' coupled with 'nothing in, nothing out' as well. Fix those data pipelines, people!
And hey, while you're at it, brush up on your threat modeling – it's a critical skill that's in short supply. Threat modelling outputs offer massive promise for making good detection engineering even better in the near future.”
And hey, while you're at it, brush up on your threat modeling – it's a critical skill that's in short supply. Threat modelling outputs offer massive promise for making good detection engineering even better in the near future.”
Data engineering integration gap signals critical capability needs
The data reveals a disconnect between the recognized importance of data engineering skills and their integration with detection engineering teams.
52%
said data engineering is
a valuable detection engineering skill they do not have and need to develop
a valuable detection engineering skill they do not have and need to develop
Detection engineers widely recognize data engineering as a crucial missing skillset (it ranked second next to threat modeling), signaling a critical gap that could uplevel their threat detection program.
39%
considered data engineering
a primary detection engineering activity at their organization
a primary detection engineering activity at their organization
Less than half of organizations listed data engineering for security as a primary activity related to detection engineering at their current place of work. In fact, it was the lowest reported detection engineering activity out of the 10 they were able to select.
35%
said there is limited or zero integration with the data engineering team
This low collaboration rate between detection and data engineering teams points to persistent organizational silos that could compromise data quality and access to necessary logging for effective threat detection programs.
“Having a dedicated detection engineering team with a priority of automation is key. It allows for scaling technology stacks to align with current and future threats.
Additionally, collaboration between detection and data engineering should be a priority. DE teams are usually limited to the log visibility available to them, so it's vital to the success of the team to maintain a strong relationship with the data engineering/log platform team.
By bridging these gaps through automation and common data standards, detection engineering teams can scale capabilities and increase detection coverage across the entire organization.”
Additionally, collaboration between detection and data engineering should be a priority. DE teams are usually limited to the log visibility available to them, so it's vital to the success of the team to maintain a strong relationship with the data engineering/log platform team.
By bridging these gaps through automation and common data standards, detection engineering teams can scale capabilities and increase detection coverage across the entire organization.”
“Much of a detection engineer's time is spent fine-tuning detections to filter out benign and expected activity. This is a tedious process required to craft high-fidelity detections. Filtering irrelevant fields and records at ingestion vs. in-query saves engineering time, reduces SIEM costs, and minimizes false positives. Pre-filtering and transforming data prior to analysis lets teams focus on higher-value tasks without the traditional trade-offs of cost, data bloat and ever-increasing query times.”
Chapter 6
The future of detection engineering
Detection engineering stands at the cusp of transformation, with artificial intelligence and automation reshaping how security teams develop, implement, and maintain detection capabilities.
88% believe AI will impact detection engineering in the next 3 years
This overwhelming consensus aligns with reality—AI isn’t just hype in detection engineering, it’s becoming table stakes. Teams should start building their AI roadmap to stay ahead of the game. That said, detection engineers don’t buy into the black-box AI of the past—those hard-to-customize, false positive-prone solutions felt more like snake oil. And no, AI isn’t replacing detection engineers. Instead, it’s here to help them work faster by taking over the least-loved tasks, so they can focus on more higher ROI activities.
Do you feel that AI will have an impact on detection engineering over the next 3 years?
Agree or
Strongly Agree
Strongly Agree
Disagree or Strongly Disagree
45% are using AI in their detection engineering efforts today
Less than half of teams have jumped into AI, showing there’s still plenty of room for thoughtful, measured adoption rather than rushing in. Security teams have been burned in the past by AI-hype from security vendors. One could argue that this data reflects that history and matches the pragmatic approach of starting small and scaling strategically.
Are you using AI in your detection engineering efforts?
No
Yes
Unknown/Unsure
Different ways detection engineers are using AI
43% of detection engineers are using AI primarily for anomaly detection, while the remaining applications are fairly evenly distributed across rule generation (20%), alert triage (19%), and other use cases (18%), highlighting the different ways AI is being integrated into detection engineering workflows.
How are you using AI?
Anomaly detection
Rule generation
Alert triage
Other
“There are few categories within cyber security that will benefit from the ai/ml/llm revolution as detection engineering, which is primed to see orders of magnitude improvements in capability in the coming years which will have a profound impact on outcomes in cyber.”
93% are using or plan to use automation in their detection engineering workflow
A significant majority of organizations are either already using automation in their detection engineering workflows or planning to implement it, with nearly two-thirds (63%) having automation already in place, indicating a strong industry-wide shift toward automation detection engineering processes.
Are you using or planning to use automation in your detection engineering workflow?
Using or planning to implement
automation
automation
Top four areas currently automated versus planning to automate
58% have implemented SOAR capabilities, the highest response to the areas teams have automated. Developing detection rules and data engineering for security rank as the top planned automation priorities at 44% each. A close second goes to tuning existing detections and threat hunting at 42%.
Which areas have you automated?
What areas are you planning to automate?
What areas are you planning to automate?
Current
58%
Automation & orchestration
50%
Threat intelligence integration
48%
Incident response
48%
Metrics and reporting
37%
Tuning existing detections
36%
Security tool administration
35%
Threat hunting
30%
Developing detection rules
25%
Data engineering for security
20%
Training and knowledge sharing
2%
Other
Planned
44%
Developing detection rules
44%
Data engineering for security
42%
Tuning existing detections
42%
Threat hunting
42%
Training and knowledge sharing
37%
Threat intelligence integration
36%
Metrics and reporting
33%
Incident response
33%
Security tool administration
31%
Automation & orchestration
9%
Other
How will detection engineering change in the future?
To answer this question, we asked our survey respondents to anonymously share their insights—no names, just honest takes on the shifts they’ve seen and what the future holds. But we also wanted to highlight the voices of security leaders shaping the future of detection engineering in public. Their firsthand experiences offer a unique window into where the field is headed. Let’s dive into both perspectives.
“Detection engineering has changed from a few ad hoc processes to a fully recognized engineering discipline. I think detection engineering will continue to evolve to build out more automation and orchestration as well as integrate with AI agents for rule tuning, development, and testing.”
“Detection engineering has changed over the last few years because it has become much more of a focus for organizations. It is critically important to understand your data, build accurate detections, and test/automate those detections to ensure they work as expected. I see this field continuing to change rapidly over the next few years as more time and energy are invested into DE efforts to automate detections, tune them, and also increase the speed in which they're deployed.”
“I have seen a big increase in interest around data fabrics, next gen SIEMs, and data lakes. There is a heightened focus on reducing costs of log ingestion and storage. Managers are also interested in leveraging AI and ML for detection (without always understanding what is and isn't possible). I believe that my organization will attempt to get more detection value out of risk based analysis and alerting in the coming years.”
"The increase in behavior based detections has caused change. The increase of AI will continue to increase change in the detection engineering space."
"Detection Engineering now is a relevant team which has gained interest in the industry and is now a fully supported function not only this, but now the maturity of those processes and how we engineer detections has become a large focus such as CI/CD, pipelines, data, quality, and governance and automated detection feedback."
"There was a shift from mostly vendor detections to in house as well. Detection program matured with the experience on the engineers both tactically when implementing a rule and strategically looking at the overall coverage. There was a lot of contribution from the incident response and SOC team. At that time there was no dedicated detection team.
As if late there has been a shift towards a separate detection team that does not have a SOC background. This raises concerns to me in their understanding of threats, elements needed for investigation and validation."
As if late there has been a shift towards a separate detection team that does not have a SOC background. This raises concerns to me in their understanding of threats, elements needed for investigation and validation."
"AI being infused for all aspects of the detection lifecycle in rapid development and testing."
"Detection as Code is the new norm, also the need to validate vendor supplied out of the box detections is becoming a requirement. For example, how do we know that [vendor] alert actually does what it says it will? If it doesn't work we're in a lot of trouble."
"The primary change I've seen is in the sheer amount of coverage needed for a modern org: endpoint, mobile, browser-based detections, and especially SaaS-based detections as well as detections related to supply-chain."
"I think AI will play a major role in recommending detections & reducing the time rules are researched & rolled out to production."
"I believe AI will be fully integrated determining threat actors that are more than likely to attack your infrastructure, also be able to map the TA iocs to the mitre att&ck framework with just a click of a button."
"AI will be pivotal in threat detection, giving teams a significant lead against even the most sophisticated attackers."
“With increased adoption of cloud, the threat landscape will continue to evolve . As a result, I anticipate that the need for a hybrid security lakes strategy will grow as it is costly and not optimal to get data out of cloud and ingest it into a single SIEM solution. There, Detection as a Service type platforms and solutions are likely to become more prominent. These solutions can support multiple cloud-native data lakes and enable organisations to perform queries directly at the source.”
“Much greater emphasis on custom rules that are intelligence-led and validated against realised threats. Looking ahead, AI will substantially increase productivity in relation to detection development.”
“For us, we have now earned the respect of the community and this was huge. Now, when we make recommendations for data components/telemetry needed for detection, we aren't met with the same resistance but rather willingness. I think awareness is key here. With awareness you can garner the support needed to obtain the data needed, in order to perform effective detection. The community is also maturing around this discipline and a lot more resources exist now than did 3 years ago. A lot of public repos with query logic, vendors giving away security tools for free/query logic, etc. Also, MITRE is quite key here with regards to threat modeling and mapping data feeds to needed data components.”
"The intent is still to detect threats operating across your environment to engage response. That being said, I believe it has been maturing over the last 3 years as we no longer want to simply cut alerts without context for every little thing but instead want highly correlated contextualized / enriched alerts with greater fidelity routing to an IR team.
It will continue maturing taking advantage of technological leaps. AI will continue to be pivotal in automating portions of the pipeline and reducing human burden for developing high fidelity detections."
It will continue maturing taking advantage of technological leaps. AI will continue to be pivotal in automating portions of the pipeline and reducing human burden for developing high fidelity detections."
"Increased training requirements for engineers to stay ahead including researcher level skillsets."
"I do think within the ranks AI will grow on detection engineering, and I do think that organizations are going to become either detection consumers or detection engineers which will change the markets and the spectrums of operations within DE"
"As the threat landscape grows more complex, there could be a push towards solutions that can correlate signals and events across network, cloud, endpoint, and other security domains. This integrated approach may provide security teams with a more comprehensive view of potential risks."
"Detection Engineering function has been unable to mature due to a constant cycle of tool migration as well as the decentralization of security data platforms (SIEMs). As AI matures, we look to improve the speed at which we can build custom detection rules as well as improve our ability to manage detection content across a distributed security landscape."
"Likely to start using "de-coupled SIEM" (hate that language) and more traction around using datalakes and things like Snowflake or Cribl Search to send high volume low impact log sources like VPC Flow to less expensive solutions."
"Detection engineering played a significant role in proactive cyber defences strategies of an organisation. Over the past three years I have seen org. who worked upon detection engineering team enablement and now taking great advantages out of it as far as proactive cyber defence is concerned. In next three years DE will be more evolved with AI/GenAI and then rule logic development, automation, fine tuning, orchestration will be more convenient for the workforce in DE."
“Simply, Detection Engineering must be a critical component of your organization’s security future and strategy. In our high stakes world of cybersecurity where the impact and consequences of an attack can be life changing for our customers, Detection platforms such as Anvilogic, which are flexible and robust enable us to be proactive rather than reactive. This allows us to create flexible detection rules and models that can learn and adapt to evolving threats in a fast moving threat landscape.
Most importantly, it enables us to take the fight to the adversaries by using detection rules to identify patterns and tactics associated with malicious activity. This enables us to stop attacks earlier and keep our customers safe.”
Most importantly, it enables us to take the fight to the adversaries by using detection rules to identify patterns and tactics associated with malicious activity. This enables us to stop attacks earlier and keep our customers safe.”
“As the complexity and pace of cyber threats continue to accelerate, traditional manual and ad hoc approaches to threat detection engineering are no longer sufficient. Modern security operations teams must adopt a structured detection engineering framework that integrates a well-designed logging architecture, a robust data pipeline for threat detection, and automation for threat use case creation and testing.
Embedding purple teaming into the detection engineering process ensures continuous validation while automating the ingestion and analysis of threat intelligence enhances detection efficacy.
The future of detection engineering lies in automation, integration, and continuous adaptation to stay ahead of evolving threats.”
Embedding purple teaming into the detection engineering process ensures continuous validation while automating the ingestion and analysis of threat intelligence enhances detection efficacy.
The future of detection engineering lies in automation, integration, and continuous adaptation to stay ahead of evolving threats.”
“Over the last few years the industry at the data
& analytics layer went through the process of unbundling services to avoid vendor lock-in, and to have more control over how data is processed, normalized, and logged.
Detection Engineering will follow suit, and enter
a phase of unbundling and abstracting away from tool dependent detections. Engineering will slowly focus on codifying detections, adhering to Security Developer disciplines, testing detection efficacy on demand, and streamline delivery processes to be product agnostic. Additionally, the use of generative A.I. will become a force multiplier for detection management, development and enrichment.”
& analytics layer went through the process of unbundling services to avoid vendor lock-in, and to have more control over how data is processed, normalized, and logged.
Detection Engineering will follow suit, and enter
a phase of unbundling and abstracting away from tool dependent detections. Engineering will slowly focus on codifying detections, adhering to Security Developer disciplines, testing detection efficacy on demand, and streamline delivery processes to be product agnostic. Additionally, the use of generative A.I. will become a force multiplier for detection management, development and enrichment.”
“Detection Engineering is a subset of security engineering that has experienced exponential growth recently and is going to continue to grow. Having proper detections based off the threat landscape of your environment can provide you with a level of assurance that you would catch suspicious or malicious activity. This of course is fueled by having enough access to log sources and traffic within your environment to give you the visibility to create rules on. It will also be excellerated by AI technology that will help generate detections on new and emerging threats.
One challenge for security teams will be to ensure testing and tuning is completed for efficiency to mitigate the risk of alert fatigue and to ensure processes are in place to take repeatable actions on the detections that are triggered.”
One challenge for security teams will be to ensure testing and tuning is completed for efficiency to mitigate the risk of alert fatigue and to ensure processes are in place to take repeatable actions on the detections that are triggered.”
“Over the past three years, advancements have been made to move off of single alert detections to series of alerts or threat scenarios. The challenge is these are still very static in nature. The future of Detection Engineering is moving towards dynamic detections with the help of AI, whether modifying criticality or dynamically chaining a series of events to streamline the alerts your analysts need to focus on with less headcount.”
Actionable Takeaways
The 2025 State of Detection Engineering report reveals a field gaining serious momentum, backed by strong leadership support and investment. While behavior-based and custom detections are on the rise to combat false positives, teams still wrestle with data access challenges, skills gaps, manual processes, and the ongoing need to communicate detection engineering’s value to leadership.
Here are four key recommendations to help you navigate this evolution and maximize your detection engineering investments:
Here are four key recommendations to help you navigate this evolution and maximize your detection engineering investments:
01
Detection engineering is getting funded, now make it count.
80% of organizations in our survey are investing in detection engineering, yet many leaders still don’t fully understand its value. Without clear communication of its impact, it risks being underutilized or deprioritized. The ROI is there—now it’s up to detection engineering teams to communicate the value up and down the org chart—and recognize the people making it happen.
Detection engineering isn’t just a technical function—it’s a strategic business enabler with metrics being reported at the board level. It plays a direct role in reducing risk, supporting compliance, improving incident response, and strengthening resilience as an organization’s environment becomes more complex with cloud adoption, AI, and emerging technologies. Failing to adequately invest in detection engineering has real downstream consequences—missing, overtuned, or broken rules can lead to a breach, and noisy detections lead to alert fatigue, increased SOC workload, and higher staffing costs just to keep up with investigations and response.
Detection engineering teams need to speak the language of impact using meaningful metrics that reflect your unique environment and threat priorities. Bridging the gap between technical execution and business objectives is key, positioning detection engineering as a driver of SOC efficiency, cost savings, and risk reduction. Tracking progress quarter over quarter and year over year is essential to demonstrating value. But let’s be honest—“metrics and reporting” ranked as the least favorite task among detection engineers. The good news? With the right detection engineering tools, much of this can be automated.
Detection engineering isn’t just a technical function—it’s a strategic business enabler with metrics being reported at the board level. It plays a direct role in reducing risk, supporting compliance, improving incident response, and strengthening resilience as an organization’s environment becomes more complex with cloud adoption, AI, and emerging technologies. Failing to adequately invest in detection engineering has real downstream consequences—missing, overtuned, or broken rules can lead to a breach, and noisy detections lead to alert fatigue, increased SOC workload, and higher staffing costs just to keep up with investigations and response.
Detection engineering teams need to speak the language of impact using meaningful metrics that reflect your unique environment and threat priorities. Bridging the gap between technical execution and business objectives is key, positioning detection engineering as a driver of SOC efficiency, cost savings, and risk reduction. Tracking progress quarter over quarter and year over year is essential to demonstrating value. But let’s be honest—“metrics and reporting” ranked as the least favorite task among detection engineers. The good news? With the right detection engineering tools, much of this can be automated.
02
Bad data equals bad detections. Fix your data problem.
Detection engineering is only as effective as the data it runs on, but nearly half report inadequate access to the data they need. For enterprise organizations, the challenge is even greater—58% lack access or aren’t sure if they have the necessary logging to meet their detection objectives. Log storage costs, inconsistent retention policies, and fragmented data creates trade-offs that leave security teams struggling to balance cost with visibility. And siloed teams can create political red tape making it difficult for detection engineers to get access to the data they need.
If you’re serious about advanced threat detection, work closely with your data engineering and application teams to ensure the right logs are gathered, normalized, and readily available. Remove any unnecessary friction put on your security teams when trying to get their hands on the data they need to meet their detection objectives. This doesn’t necessarily mean more logs but better-managed, purposeful data that fuels accurate detections unique to your environment. It’s 2025, if legacy log management architectures are holding you back due to rising costs, investigate supplementing them by putting high volume data feeds in more cost effective security data lakes that are likely already being used by other teams at your organization.
If you’re serious about advanced threat detection, work closely with your data engineering and application teams to ensure the right logs are gathered, normalized, and readily available. Remove any unnecessary friction put on your security teams when trying to get their hands on the data they need to meet their detection objectives. This doesn’t necessarily mean more logs but better-managed, purposeful data that fuels accurate detections unique to your environment. It’s 2025, if legacy log management architectures are holding you back due to rising costs, investigate supplementing them by putting high volume data feeds in more cost effective security data lakes that are likely already being used by other teams at your organization.
03
Build the skills that will shape the future of detection engineering.
Detection engineering teams aren’t just battling threats—they’re navigating data overload, outdated processes, and the need to deeply understand their unique environment to better protect their organization. The shift from tactical detections to behavior-based detection strategies is being led by highly skilled teams tackling increasingly sophisticated threats. As they refine their expertise, they’re not just improving their own capabilities—they’re pushing the entire industry forward!
A behavior-based detection strategy requires understanding of how adversaries operate and where their digital footprints show up. According to our survey, teams recognize key skill gaps that could dramatically improve their detection capabilities:.
A behavior-based detection strategy requires understanding of how adversaries operate and where their digital footprints show up. According to our survey, teams recognize key skill gaps that could dramatically improve their detection capabilities:.
- Threat modeling (53%): Collaborating cross-functionally to map out potential attack paths for your highest priority threats before they happen helps teams anticipate adversary movements and refine detection strategies.
- Data engineering (52%): Understanding what data to collect, filter, and enrich ensures security teams get the right telemetry at the right time—without drowning in noise. This skillset also helps security teams better collaborate with external teams who hold access to the logging they need to be successful.
- Software development life cycle principles (47%) and detection-as-code (46%): These skills go hand in hand. As detection engineering evolves, teams are increasingly adopting version control, structured workflows, and reusable detection logic to keep rules efficient, consistent, and adaptable when managing hundreds or thousands of detections across diverse data platforms and security tools.
04
Put generative AI and automation to work, while keeping the human touch.
Automation is thriving in detection engineering, but AI adoption is still catching up. 88% of security teams believe AI will have a massive impact on detection engineering by 2028, but only 45% are actually using it today. We know AI hype is everywhere, with some vendors even promising a fully autonomous SOC. Let’s be clear—AI won’t replace detection engineers. But it can scale their expertise, improve team collaboration, and help them work faster. Tasks that once took hours like collecting, processing and comprehending data can now be done in minutes with generative AI (GenAI).
AI copilots act as real-time assistants, offering query recommendations, threat insights, and context-aware analysis to accelerate investigations. GenAI can streamline data normalization, ensuring cleaner, more structured data feeds for accurate detections. It can also automate documentation and runbook creation, providing SOC analysts with clear, consistent investigative guidance. Beyond efficiency, AI can help detection engineers expand their expertise—enabling specialists in one domain, like endpoint security, to more easily build detections for new areas such as cloud security. Now is the time to explore how GenAI can support and elevate your detection engineering strategy. The key is thoughtful implementation to remove bottlenecks and free up teams to focus on more complex tasks, ultimately enhancing the effectiveness of their detection engineering programs.
AI copilots act as real-time assistants, offering query recommendations, threat insights, and context-aware analysis to accelerate investigations. GenAI can streamline data normalization, ensuring cleaner, more structured data feeds for accurate detections. It can also automate documentation and runbook creation, providing SOC analysts with clear, consistent investigative guidance. Beyond efficiency, AI can help detection engineers expand their expertise—enabling specialists in one domain, like endpoint security, to more easily build detections for new areas such as cloud security. Now is the time to explore how GenAI can support and elevate your detection engineering strategy. The key is thoughtful implementation to remove bottlenecks and free up teams to focus on more complex tasks, ultimately enhancing the effectiveness of their detection engineering programs.
Final thoughts
Our first-ever State of Detection Engineering report reveals mounting pressures on detection engineering teams as their role becomes mission-critical.
Anvilogic’s detection engineering platform helps teams overcome many of these challenges by giving them the freedom to build and scale detections across their security stack—without vendor lock-in or data silos. Whether deploying behavioral-based detections in a SIEM, data lake, or both, teams no longer have to choose between data visibility and cost. With Anvilogic, security teams can measure and align detection coverage to their unique threat priorities, build correlated detections tailored to their environment to tackle false positives, and reduce manual maintenance with a security copilot that helps them build, tune, and fix broken rules. Trusted by leading enterprise security teams, Anvilogic helps organizations detect threats at scale and save millions of dollars.
Learn how Anvilogic puts AI and automation to work for your detection engineering team.
Anvilogic’s detection engineering platform helps teams overcome many of these challenges by giving them the freedom to build and scale detections across their security stack—without vendor lock-in or data silos. Whether deploying behavioral-based detections in a SIEM, data lake, or both, teams no longer have to choose between data visibility and cost. With Anvilogic, security teams can measure and align detection coverage to their unique threat priorities, build correlated detections tailored to their environment to tackle false positives, and reduce manual maintenance with a security copilot that helps them build, tune, and fix broken rules. Trusted by leading enterprise security teams, Anvilogic helps organizations detect threats at scale and save millions of dollars.
Learn how Anvilogic puts AI and automation to work for your detection engineering team.