By: Kevin Gonzalez, VP of Security, Operations, and Data at Anvilogic
The emergence of Artificial Intelligence (AI) has fundamentally changed the landscape of cybersecurity. Threat actors are becoming more versatile, utilizing AI-driven tools to develop sophisticated attack methods that bypass traditional defenses. The complexity of modern threats demands an evolution in detection engineering that provides an effective way to improve and escalate detections. This approach should leverage data science and machine learning techniques to address the scale and intricacy of the challenges posed by today's advanced threats.
This is where the Detection Engineering Escalation & Recommendation (DEER) Framework comes into play–a new paradigm designed to transform how we approach Security Operations Center (SOC) challenges.
The Need for a New Detection Paradigm
For years, detection engineering has relied on static rules and signatures–an approach that is, at best, reactive. When a new threat emerges, detection engineers scramble to create indicators of attack (IoAs) or indicators of compromise (IoCs) and tune their rules to catch suspicious activities. The result is often a barrage of alerts, many adding another layer of noise that needs to be managed rather than help identify the actual threats. This traditional model burdens SOC analysts heavily, leading to burnout, alert fatigue, and a decreased ability to detect and respond to actual incidents effectively.
Moreover, attempts to introduce machine learning (ML) into cybersecurity have often been focused on replacing the detection engineer entirely. These efforts concentrate on processing raw telemetry data to identify suspicious activities without adequately considering context or integrating human expertise into the process. Although they may be experts in their fields, data scientists may not have the security operations domain expertise necessary to identify real threats and translate their findings into actionable insights for incident response teams to triage. This disconnect has led to noisy signals that add little value in real-world scenarios.
Introducing the Detection Engineering Escalation and Recommendation (DEER) Framework
The DEER framework seeks to address these detection engineering challenges by shifting the role of the data scientist to where it can have the most impact: on standardized context-rich datasets. This approach emphasizes the necessity of a foundational detection engineering practice where atomic behavioral detections are purpose-built with proper enrichment tagging and move data science away from operating on raw telemetry data towards the outputs of these detections. By focusing on this shift, detection engineers can do what they do best, engineering detection content purpose-built for threats, and data science can take place where it is most effective–on top of structured data sets, leading to an effective means of tuning detections, identifying threats, streamlining response, and reducing overall analyst workload.
The DEER framework leverages a melting pot of data science concepts supported by cybersecurity expertise, including:
- Natural Language Processing (NLP) for Entity Extraction and Embeddings: Alerts often contain additional data points, such as IP addresses in command line strings, that are not always extracted during detection. NLP techniques ensure that all relevant entities are accounted for, allowing for easy IoA or IoC lookups against threat intelligence sources. This provides a richer context for analysis and facilitates better decision-making.
- Learning-to-Rank (LTR) for Alert Prioritization: Effective SOC operations hinge on accurately prioritizing alerts. By leveraging LTR models on top of detection outputs, we can integrate analyst feedback directly into alert prioritization. This dynamic feedback loop ensures that high-priority alerts are surfaced first, reducing the risk of missing critical threats while continuously improving the relevance of alerts over time.
- Large Language Models (LLMs) for Explainability, Analysis, Tuning, and Escalation: The rise of LLMs offers significant potential for defenders despite adversaries’ misuse. A considerable portion of alert triage involves understanding and profiling alert contents. LLMs can help accelerate this process by explaining why an alert was triggered and providing actionable remediation recommendations. This capability significantly reduces the response time and enhances the efficiency of the SOC.
- Clustering for Enhanced Context and Expandability: Traditional clustering techniques applied to raw telemetry often resulted in overwhelming and context-poor alerts, leaving analysts with time-consuming investigations. By applying clustering to detection engineering outputs, data scientists can surface unusual sequences within context-rich alerts, leading to higher fidelity threat detections. This approach allows triage analysts to see the entire attack chain, providing a more comprehensive understanding of the threat rather than having to piece together disparate events.
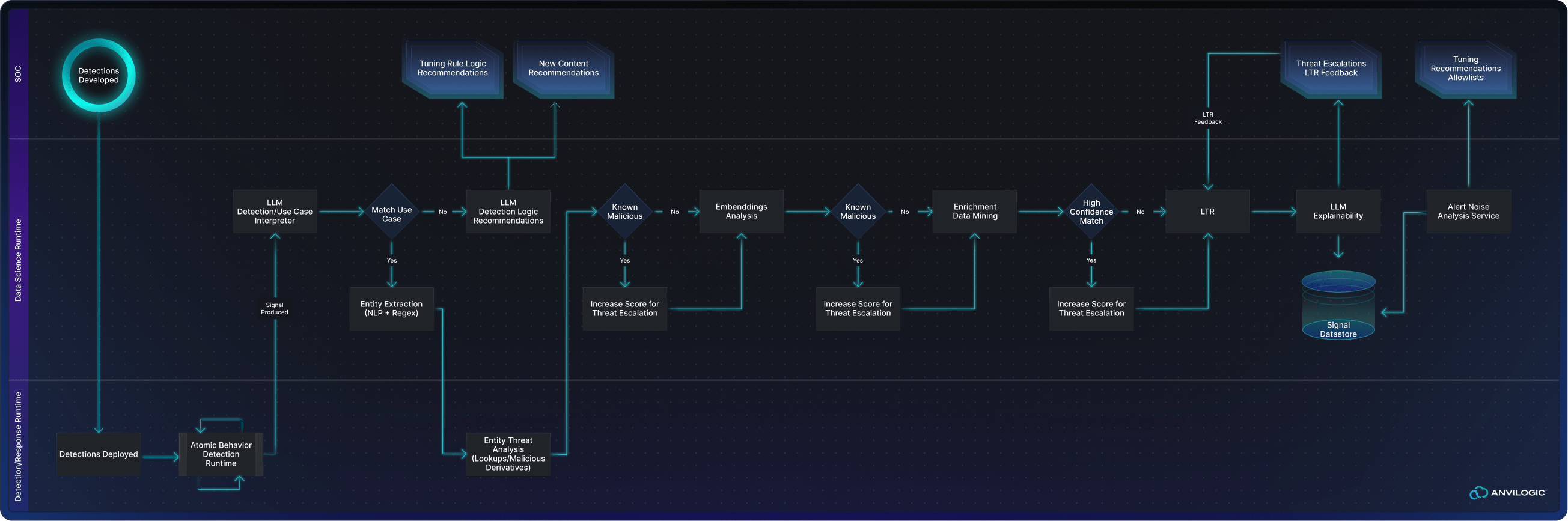
Download this diagram as a PDF
Creating Synergy Across Teams
Shifting data science efforts to post-signal processing and highlighting the importance of detection engineering practices shifts the collaborative relationships between threat researchers, detection engineers, threat hunters, and data scientists. Each team plays a crucial role in ensuring the success of the DEER framework, contributing their expertise and collaborating to enhance the overall SOC process. A key thing to note is that these teams report to the SOC function; they are not decentralized under different reporting structures and priorities.
- Threat Research Team: The threat research team generates the foundational content used throughout the pipeline. This content is surfaced to detection engineers, threat hunters, and data scientists. Detection engineers use it to build detection logic, threat hunters leverage it for post-compromise checks, and the data science team uses it as consumable data to power detection models.
- Detection Engineering Team: The detection engineering team takes the content produced by the threat research team and translates it into atomic-level detections. These detections produce signals, which are then fed into an alert lake. This alert lake becomes the centralized repository that serves as the foundation for data science and hunting efforts.
- Threat Hunting Team: Typically composed of senior-level SOC personnel, the threat hunting team focuses on post-compromise checks against raw telemetry based on threat research and feeds findings to detection engineering for high-quality detection outputs. They conduct deep investigations using the data stored in the alert lake to identify potential compromises that may have bypassed automated detections. The threat hunters provide feedback to both the detection engineering and data science teams, enabling continuous improvement of detection logic, enrichment, and models–such as LTR.
- Data Science Team: The data science team is embedded within the broader SOC team, enabling close collaboration. They leverage feedback loops from threat-hunting activities to develop and train models more adept at detecting threats. They also use feedback from the detection engineering team to analyze alerts and enhance tuning models effectively. By consuming the content produced by the threat research and detection engineering team, data scientists build models that understand the nature of threats and identify similar activities in the environment. Their final output is a fully explained and expanded-upon alert ready for response.
The result of this team structure and flow are two-fold: Intelligent Detection Escalations and Tuning Recommendations.
Strategic Benefits for Security Leadership
For CISOs and Security Architects, the DEER paradigm represents a shift from reactive detection to proactive, intelligent detection and threat management. Instead of relying on outdated static rules or noisy machine learning models that operate on raw telemetry, the DEER approach focuses on foundational detection engineering practices. It integrates data science downstream–where it is most effective. This alignment reduces the workload on detection engineers and SOC analysts and creates a feedback loop that continuously refines detection capabilities.
The strategic benefits of the DEER framework include:
- Reduced Alert Fatigue: By automating the tuning of detection logic and incorporating real-time feedback, the DEER pipeline significantly reduces false positives, allowing SOC analysts to focus on genuine threats.
- Enhanced Detection Precision: Behavioral-based detections and data science models, such as LLMs and NER, provide context-rich alerts, improving the accuracy and relevance of detections.
- Increased Operational Efficiency: Automating tedious detection engineering and triage tasks frees up valuable analyst time, allowing them to concentrate on higher-level activities.
- Continuous Improvement: Embedding data science models into the detection process ensures that your detection engineering process evolves with the threat landscape. This continuous improvement is vital in maintaining a resilient security posture.
Embracing the DEER Paradigm for Proactive, Intelligence-Driven Security
Detection Engineering Escalation and Recommendation (DEER) is more than just an evolution of detection engineering—it is a paradigm shift that integrates data science where it matters most, enhances detection precision, and drives strategic alignment with business objectives. By focusing on behavioral detections and leveraging advanced data science techniques, the DEER pipeline transforms the SOC from a reactive center of alert triage to a proactive, intelligence-driven hub.
The DEER framework is built on collaboration–bringing together threat researchers, detection engineers, threat hunters, and data scientists to create a unified approach to security. Each role has a critical part to play, and their collaboration ensures that detection is precise, response is swift, and continuous improvement is a core part of the process.
As cyber threats continue to evolve, security teams must evolve, too. The DEER framework empowers SOCs to not only keep pace but also gain the upper hand. By leveraging the same advanced data techniques adversaries often exploit, DEER enables security teams to turn the tables on attackers. The result? A SOC that is more resilient, efficient, and capable of addressing the challenges of modern cybersecurity.
Check out our solution guide to learn how Anvilogic can help detection engineers augment their existing SIEM deployments to build more accurate detections and hunt more effectively.